目錄
- 一、概述
- 二、匹配關鍵詞
- 三、算法實現(xiàn)
- 3.1、構建存儲結構
- 3.2、匹配關鍵詞
- 3.3、完整代碼
- 四、其他用法
一、概述
計算機操作系統(tǒng)中的進程狀態(tài)與切換可以作為 DFA 算法的一種近似理解。如下圖所示,其中橢圓表示狀態(tài),狀態(tài)之間的連線表示事件,進程的狀態(tài)以及事件都是可確定的,且都可以窮舉。

DFA 算法具有多種應用,在此先介紹在匹配關鍵詞領域的應用。
二、匹配關鍵詞
我們可以將每個文本片段作為狀態(tài),例如“匹配關鍵詞”可拆分為“匹”、“匹配”、“匹配關”、“匹配關鍵”和“匹配關鍵詞”五個文本片段。

【過程】:
- 初始狀態(tài)為空,當觸發(fā)事件“匹”時轉換到狀態(tài)“匹”;
- 觸發(fā)事件“配”,轉換到狀態(tài)“匹配”;
- 依次類推,直到轉換為最后一個狀態(tài)“匹配關鍵詞”。
再讓我們考慮多個關鍵詞的情況,例如“匹配算法”、“匹配關鍵詞”以及“信息抽取”。
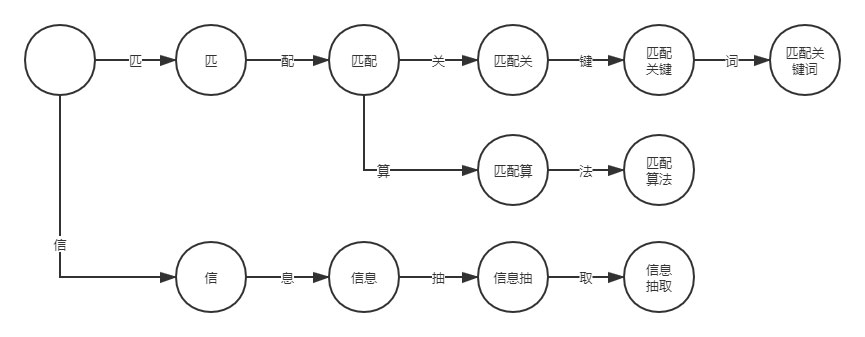
可以看到上圖的狀態(tài)圖類似樹形結構,也正是因為這個結構,使得 DFA 算法在關鍵詞匹配方面要快于關鍵詞迭代方法(for 循環(huán))。經常刷 LeetCode 的讀者應該清楚樹形結構的時間復雜度要小于 for 循環(huán)的時間復雜度。
for 循環(huán):
keyword_list = []
for keyword in ["匹配算法", "匹配關鍵詞", "信息抽取"]:
if keyword in "DFA 算法匹配關鍵詞":
keyword_list.append(keyword)
for 循環(huán)需要遍歷一遍關鍵詞表,隨著關鍵詞表的擴充,所需的時間也會越來越長。
DFA 算法:找到“匹”時,只會按照事件走向特定的序列,例如“匹配關鍵詞”,而不會走向“匹配算法”,因此遍歷的次數要小于 for 循環(huán)。具體的實現(xiàn)放在下文中。
【問】:那么如何構建狀態(tài)圖所示的結構呢?
【答】:在 Python 中我們可以使用 dict 數據結構。
state_event_dict = {
"匹": {
"配": {
"算": {
"法": {
"is_end": True
},
"is_end": False
},
"關": {
"鍵": {
"詞": {
"is_end": True
},
"is_end": False
},
"is_end": False
},
"is_end": False
},
"is_end": False
},
"信": {
"息": {
"抽": {
"取": {
"is_end": True
},
"is_end": False
},
"is_end": False
},
"is_end": False
}
}
用嵌套字典來作為樹形結構,key 作為事件,通過 is_end
字段來判斷狀態(tài)是否為最后一個狀態(tài),如果是最后一個狀態(tài),則停止狀態(tài)轉換,獲取匹配的關鍵詞。
【問】:如果關鍵詞存在包含關系,例如“匹配關鍵詞”和“匹配”,那么該如何處理呢?
【答】:我們仍然可以用 is_end
字段來表示關鍵詞的結尾,同時添加一個新的字段,例如 is_continue
來表明仍可繼續(xù)進行匹配。除此之外,也可以通過尋找除 is_end
字段外是否還有其他的字段來判斷是否繼續(xù)進行匹配。例如下面代碼中的“配”,除了 is_end
字段外還有“關”,因此還需要繼續(xù)進行匹配。
state_event_dict = {
"匹": {
"配": {
"關": {
"鍵": {
"詞": {
"is_end": True
},
"is_end": False
},
"is_end": False
},
"is_end": True
},
"is_end": False
}
}
接下來,我們來實現(xiàn)這個算法。
三、算法實現(xiàn)
使用 Python 3.6 版本實現(xiàn),當然 Python 3.X 都能運行。
3.1、構建存儲結構
def _generate_state_event_dict(keyword_list: list) -> dict:
state_event_dict = {}
# 遍歷每一個關鍵詞
for keyword in keyword_list:
current_dict = state_event_dict
length = len(keyword)
for index, char in enumerate(keyword):
if char not in current_dict:
next_dict = {"is_end": False}
current_dict[char] = next_dict
current_dict = next_dict
else:
next_dict = current_dict[char]
current_dict = next_dict
if index == length - 1:
current_dict["is_end"] = True
return state_event_dict
關于上述代碼仍然有不少可迭代優(yōu)化的地方,例如先對關鍵詞列表按照字典序進行排序,這樣可以讓具有相同前綴的關鍵詞集中在一塊,從而在構建存儲結構時能夠減少遍歷的次數。
3.2、匹配關鍵詞
def match(state_event_dict: dict, content: str):
match_list = []
state_list = []
temp_match_list = []
for char_pos, char in enumerate(content):
# 首先找到匹配項的起點
if char in state_event_dict:
state_list.append(state_event_dict)
temp_match_list.append({
"start": char_pos,
"match": ""
})
# 可能會同時滿足多個匹配項,因此遍歷這些匹配項
for index, state in enumerate(state_list):
if char in state:
state_list[index] = state[char]
temp_match_list[index]["match"] += char
# 如果抵達匹配項的結尾,表明匹配關鍵詞完成
if state[char]["is_end"]:
match_list.append(copy.deepcopy(temp_match_list[index]))
# 如果還能繼續(xù),則繼續(xù)進行匹配
if len(state[char].keys()) == 1:
state_list.pop(index)
temp_match_list.pop(index)
# 如果不滿足匹配項的要求,則將其移除
else:
state_list.pop(index)
temp_match_list.pop(index)
return match_list
3.3、完整代碼
import re
import copy
class DFA:
def __init__(self, keyword_list: list):
self.state_event_dict = self._generate_state_event_dict(keyword_list)
def match(self, content: str):
match_list = []
state_list = []
temp_match_list = []
for char_pos, char in enumerate(content):
if char in self.state_event_dict:
state_list.append(self.state_event_dict)
temp_match_list.append({
"start": char_pos,
"match": ""
})
for index, state in enumerate(state_list):
if char in state:
state_list[index] = state[char]
temp_match_list[index]["match"] += char
if state[char]["is_end"]:
match_list.append(copy.deepcopy(temp_match_list[index]))
if len(state[char].keys()) == 1:
state_list.pop(index)
temp_match_list.pop(index)
else:
state_list.pop(index)
temp_match_list.pop(index)
return match_list
@staticmethod
def _generate_state_event_dict(keyword_list: list) -> dict:
state_event_dict = {}
for keyword in keyword_list:
current_dict = state_event_dict
length = len(keyword)
for index, char in enumerate(keyword):
if char not in current_dict:
next_dict = {"is_end": False}
current_dict[char] = next_dict
current_dict = next_dict
else:
next_dict = current_dict[char]
current_dict = next_dict
if index == length - 1:
current_dict["is_end"] = True
return state_event_dict
if __name__ == "__main__":
dfa = DFA(["匹配關鍵詞", "匹配算法", "信息抽取", "匹配"])
print(dfa.match("信息抽取之 DFA 算法匹配關鍵詞,匹配算法"))
輸出:
[
{
'start': 0,
'match': '信息抽取'
}, {
'start': 12,
'match': '匹配'
}, {
'start': 12,
'match': '匹配關鍵詞'
}, {
'start': 18,
'match': '匹配'
}, {
'start': 18,
'match': '匹配算法'
}
]
四、其他用法
4.1、添加通配符
在敏感詞識別時往往會遇到同一種類型的句式,例如“你這個傻X”,其中 X 可以有很多,難道我們需要一個個添加到關鍵詞表中嗎?最好能夠通過類似正則表達式的方法去進行識別。一個簡單的做法就是“*”,匹配任何內容。
添加通配符只需要對匹配關鍵詞過程進行修改:
def match(self, content: str):
match_list = []
state_list = []
temp_match_list = []
for char_pos, char in enumerate(content):
if char in self.state_event_dict:
state_list.append(self.state_event_dict)
temp_match_list.append({
"start": char_pos,
"match": ""
})
for index, state in enumerate(state_list):
is_find = False
state_char = None
# 如果是 * 則匹配所有內容
if "*" in state:
state_list[index] = state["*"]
state_char = state["*"]
is_find = True
if char in state:
state_list[index] = state[char]
state_char = state[char]
is_find = True
if is_find:
temp_match_list[index]["match"] += char
if state_char["is_end"]:
match_list.append(copy.deepcopy(temp_match_list[index]))
if len(state_char.keys()) == 1:
state_list.pop(index)
temp_match_list.pop(index)
else:
state_list.pop(index)
temp_match_list.pop(index)
return match_list
main() 函數。
if __name__ == "__main__":
dfa = DFA(["匹配關鍵詞", "匹配算法", "信息*取", "匹配"])
print(dfa.match("信息抽取之 DFA 算法匹配關鍵詞,匹配算法,信息抓取"))
輸出:
[
{
'start': 0,
'match': '信息抽取'
}, {
'start': 12,
'match': '匹配'
}, {
'start': 12,
'match': '匹配關鍵詞'
}, {
'start': 18,
'match': '匹配'
}, {
'start': 18,
'match': '匹配算法'
}, {
'start': 23,
'match': '信息抓取'
}
]
以上就是淺析Python實現(xiàn)DFA算法的詳細內容,更多關于Python DFA算法的資料請關注腳本之家其它相關文章!
您可能感興趣的文章:- 基于java實現(xiàn)DFA算法代碼實例
- Java實現(xiàn)DFA算法對敏感詞、廣告詞過濾功能示例
- Java使用DFA算法實現(xiàn)過濾多家公司自定義敏感字功能詳解
- java利用DFA算法實現(xiàn)敏感詞過濾功能
- C#詞法分析器之轉換DFA詳解