目錄
- 一、環(huán)境準備
- 二、問題分析
- 三、spider
- 四、item
- 五、setting
- 六、pipelines
- 七、middlewares
- 八、使用jupyter進行簡單的處理和分析
一、環(huán)境準備
- python3.8.3
- pycharm
- 項目所需第三方包
pip install scrapy fake-useragent requests selenium virtualenv -i https://pypi.douban.com/simple
1.1 創(chuàng)建虛擬環(huán)境
切換到指定目錄創(chuàng)建
創(chuàng)建完記得激活虛擬環(huán)境
1.2 創(chuàng)建項目
1.3 使用pycharm打開項目,將創(chuàng)建的虛擬環(huán)境配置到項目中來
1.4 創(chuàng)建京東spider
scrapy genspider 爬蟲名稱 url
1.5 修改允許訪問的域名,刪除https:
二、問題分析
爬取數(shù)據(jù)的思路是先獲取首頁的基本信息,在獲取詳情頁商品詳細信息;爬取京東數(shù)據(jù)時,只返回40條數(shù)據(jù),這里,作者使用selenium,在scrapy框架中編寫下載器中間件,返回頁面所有數(shù)據(jù)。
爬取的字段分別是:
商品價格
商品評數(shù)
商品店家
商品SKU(京東可直接搜索到對應(yīng)的產(chǎn)品)
商品標題
商品詳細信息
三、spider
import re
import scrapy
from lianjia.items import jd_detailItem
class JiComputerDetailSpider(scrapy.Spider):
name = 'ji_computer_detail'
allowed_domains = ['search.jd.com', 'item.jd.com']
start_urls = [
'https://search.jd.com/Search?keyword=%E7%AC%94%E8%AE%B0%E6%9C%AC%E7%94%B5%E8%84%91suggest=1.def.0.basewq=%E7%AC%94%E8%AE%B0%E6%9C%AC%E7%94%B5%E8%84%91page=1s=1click=0']
def parse(self, response):
lls = response.xpath('//ul[@class="gl-warp clearfix"]/li')
for ll in lls:
item = jd_detailItem()
computer_price = ll.xpath('.//div[@class="p-price"]/strong/i/text()').extract_first()
computer_commit = ll.xpath('.//div[@class="p-commit"]/strong/a/text()').extract_first()
computer_p_shop = ll.xpath('.//div[@class="p-shop"]/span/a/text()').extract_first()
item['computer_price'] = computer_price
item['computer_commit'] = computer_commit
item['computer_p_shop'] = computer_p_shop
meta = {
'item': item
}
shop_detail_url = ll.xpath('.//div[@class="p-img"]/a/@href').extract_first()
shop_detail_url = 'https:' + shop_detail_url
yield scrapy.Request(url=shop_detail_url, callback=self.detail_parse, meta=meta)
for i in range(2, 200, 2):
next_page_url = f'https://search.jd.com/Search?keyword=%E7%AC%94%E8%AE%B0%E6%9C%AC%E7%94%B5%E8%84%91suggest=1.def.0.basewq=%E7%AC%94%E8%AE%B0%E6%9C%AC%E7%94%B5%E8%84%91page={i}s=116click=0'
yield scrapy.Request(url=next_page_url, callback=self.parse)
def detail_parse(self, response):
item = response.meta.get('item')
computer_sku = response.xpath('//a[@class="notice J-notify-sale"]/@data-sku').extract_first()
item['computer_sku'] = computer_sku
computer_title = response.xpath('//div[@class="sku-name"]/text()').extract_first().strip()
computer_title = ''.join(re.findall('\S', computer_title))
item['computer_title'] = computer_title
computer_detail = response.xpath('string(//ul[@class="parameter2 p-parameter-list"])').extract_first().strip()
computer_detail = ''.join(re.findall('\S', computer_detail))
item['computer_detail'] = computer_detail
yield item
四、item
class jd_detailItem(scrapy.Item):
# define the fields for your item here like:
computer_sku = scrapy.Field()
computer_price = scrapy.Field()
computer_title = scrapy.Field()
computer_commit = scrapy.Field()
computer_p_shop = scrapy.Field()
computer_detail = scrapy.Field()
五、setting
import random
from fake_useragent import UserAgent
ua = UserAgent()
USER_AGENT = ua.random
ROBOTSTXT_OBEY = False
DOWNLOAD_DELAY = random.uniform(0.5, 1)
DOWNLOADER_MIDDLEWARES = {
'lianjia.middlewares.jdDownloaderMiddleware': 543
}
ITEM_PIPELINES = {
'lianjia.pipelines.jd_csv_Pipeline': 300
}
六、pipelines
class jd_csv_Pipeline:
# def process_item(self, item, spider):
# return item
def open_spider(self, spider):
self.fp = open('./jd_computer_message.xlsx', mode='w+', encoding='utf-8')
self.fp.write('computer_sku\tcomputer_title\tcomputer_p_shop\tcomputer_price\tcomputer_commit\tcomputer_detail\n')
def process_item(self, item, spider):
# 寫入文件
try:
line = '\t'.join(list(item.values())) + '\n'
self.fp.write(line)
return item
except:
pass
def close_spider(self, spider):
# 關(guān)閉文件
self.fp.close()
七、middlewares
class jdDownloaderMiddleware:
def process_request(self, request, spider):
# 判斷是否是ji_computer_detail的爬蟲
# 判斷是否是首頁
if spider.name == 'ji_computer_detail' and re.findall(f'.*(item.jd.com).*', request.url) == []:
options = ChromeOptions()
options.add_argument("--headless")
driver = webdriver.Chrome(options=options)
driver.get(request.url)
for i in range(0, 15000, 5000):
driver.execute_script(f'window.scrollTo(0, {i})')
time.sleep(0.5)
body = driver.page_source.encode()
time.sleep(1)
return HtmlResponse(url=request.url, body=body, request=request)
return None
八、使用jupyter進行簡單的處理和分析
其他文件:百度停用詞庫、簡體字文件
下載第三方包
!pip install seaborn jieba wordcloud PIL -i https://pypi.douban.com/simple
8.1導(dǎo)入第三方包
import re
import os
import jieba
import wordcloud
import pandas as pd
import numpy as np
from PIL import Image
import seaborn as sns
from docx import Document
from docx.shared import Inches
import matplotlib.pyplot as plt
from pandas import DataFrame,Series
8.2設(shè)置可視化的默認字體和seaborn的樣式
sns.set_style('darkgrid')
plt.rcParams['font.sans-serif'] = ['SimHei']
plt.rcParams['axes.unicode_minus'] = False
8.3讀取數(shù)據(jù)
df_jp = pd.read_excel('./jd_shop.xlsx')
8.4篩選Inteli5、i7、i9處理器數(shù)據(jù)
def convert_one(s):
if re.findall(f'.*?(i5).*', str(s)) != []:
return re.findall(f'.*?(i5).*', str(s))[0]
elif re.findall(f'.*?(i7).*', str(s)) != []:
return re.findall(f'.*?(i7).*', str(s))[0]
elif re.findall(f'.*?(i9).*', str(s)) != []:
return re.findall(f'.*?(i9).*', str(s))[0]
df_jp['computer_intel'] = df_jp['computer_detail'].map(convert_one)
8.5篩選筆記本電腦的屏幕尺寸范圍
def convert_two(s):
if re.findall(f'.*?(\d+\.\d+英寸-\d+\.\d+英寸).*', str(s)) != []:
return re.findall(f'.*?(\d+\.\d+英寸-\d+\.\d+英寸).*', str(s))[0]
df_jp['computer_in'] = df_jp['computer_detail'].map(convert_two)
8.6將評論數(shù)轉(zhuǎn)化為整形
def convert_three(s):
if re.findall(f'(\d+)萬+', str(s)) != []:
number = int(re.findall(f'(\d+)萬+', str(s))[0]) * 10000
return number
elif re.findall(f'(\d+)+', str(s)) != []:
number = re.findall(f'(\d+)+', str(s))[0]
return number
df_jp['computer_commit'] = df_jp['computer_commit'].map(convert_three)
8.7篩選出需要分析的品牌
def find_computer(name, s):
sr = re.findall(f'.*({name}).*', str(s))[0]
return sr
def convert(s):
if re.findall(f'.*(聯(lián)想).*', str(s)) != []:
return find_computer('聯(lián)想', s)
elif re.findall(f'.*(惠普).*', str(s)) != []:
return find_computer('惠普', s)
elif re.findall(f'.*(華為).*', str(s)) != []:
return find_computer('華為', s)
elif re.findall(f'.*(戴爾).*', str(s)) != []:
return find_computer('戴爾', s)
elif re.findall(f'.*(華碩).*', str(s)) != []:
return find_computer('華碩', s)
elif re.findall(f'.*(小米).*', str(s)) != []:
return find_computer('小米', s)
elif re.findall(f'.*(榮耀).*', str(s)) != []:
return find_computer('榮耀', s)
elif re.findall(f'.*(神舟).*', str(s)) != []:
return find_computer('神舟', s)
elif re.findall(f'.*(外星人).*', str(s)) != []:
return find_computer('外星人', s)
df_jp['computer_p_shop'] = df_jp['computer_p_shop'].map(convert)
8.8刪除指定字段為空值的數(shù)據(jù)
for n in ['computer_price', 'computer_commit', 'computer_p_shop', 'computer_sku', 'computer_detail', 'computer_intel', 'computer_in']:
index_ls = df_jp[df_jp[[n]].isnull().any(axis=1)==True].index
df_jp.drop(index=index_ls, inplace=True)
8.9查看各品牌的平均價格
plt.figure(figsize=(10, 8), dpi=100)
ax = sns.barplot(x='computer_p_shop', y='computer_price', data=df_jp.groupby(by='computer_p_shop')[['computer_price']].mean().reset_index())
for index,row in df_jp.groupby(by='computer_p_shop')[['computer_price']].mean().reset_index().iterrows():
ax.text(row.name,row['computer_price'] + 2,round(row['computer_price'],2),color="black",ha="center")
ax.set_xlabel('品牌')
ax.set_ylabel('平均價格')
ax.set_title('各品牌平均價格')
boxplot_fig = ax.get_figure()
boxplot_fig.savefig('各品牌平均價格.png', dpi=400)
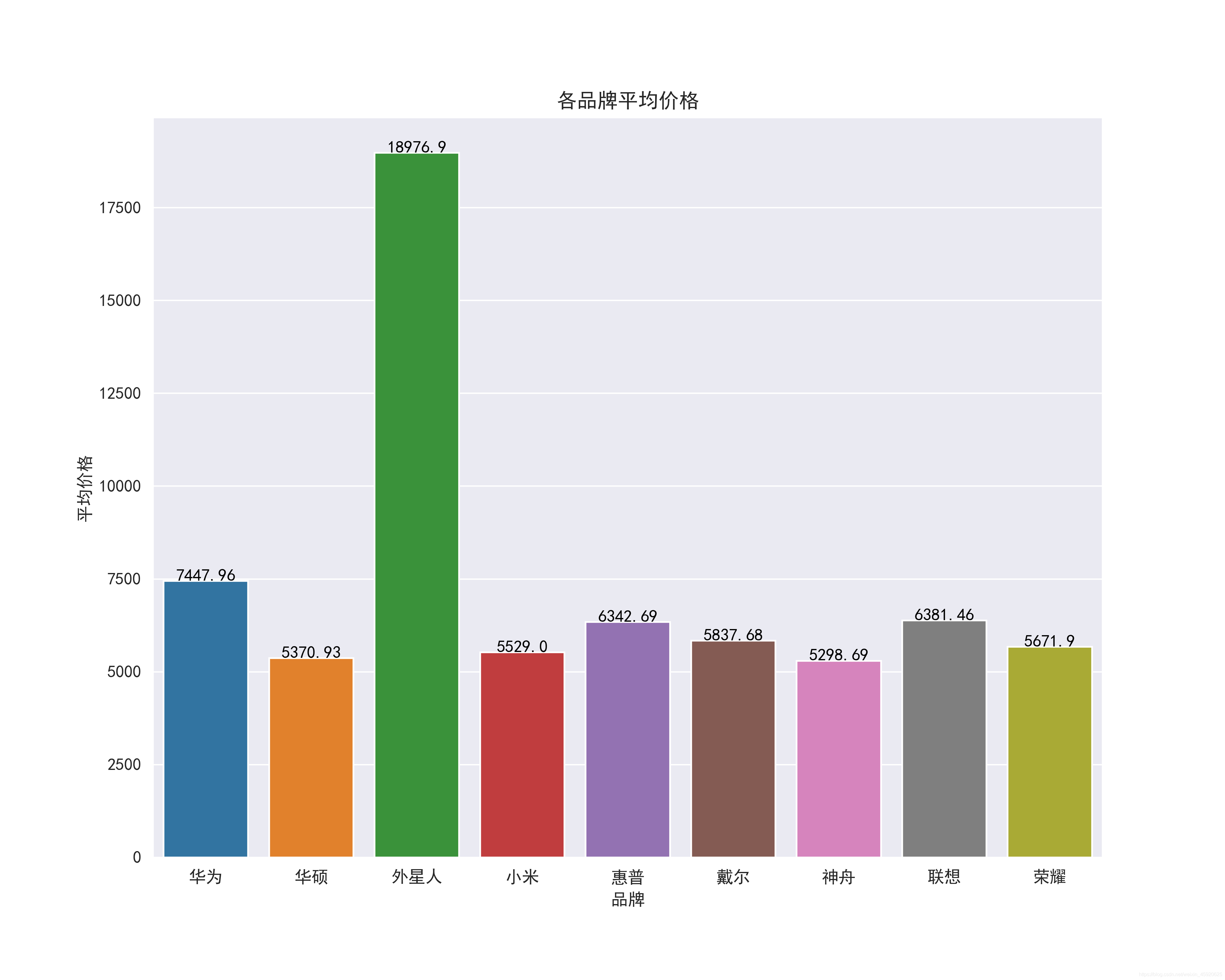
8.10 查看各品牌的價格區(qū)間
plt.figure(figsize=(10, 8), dpi=100)
ax = sns.boxenplot(x='computer_p_shop', y='computer_price', data=df_jp.query('computer_price>500'))
ax.set_xlabel('品牌')
ax.set_ylabel('價格區(qū)間')
ax.set_title('各品牌價格區(qū)間')
boxplot_fig = ax.get_figure()
boxplot_fig.savefig('各品牌價格區(qū)間.png', dpi=400)
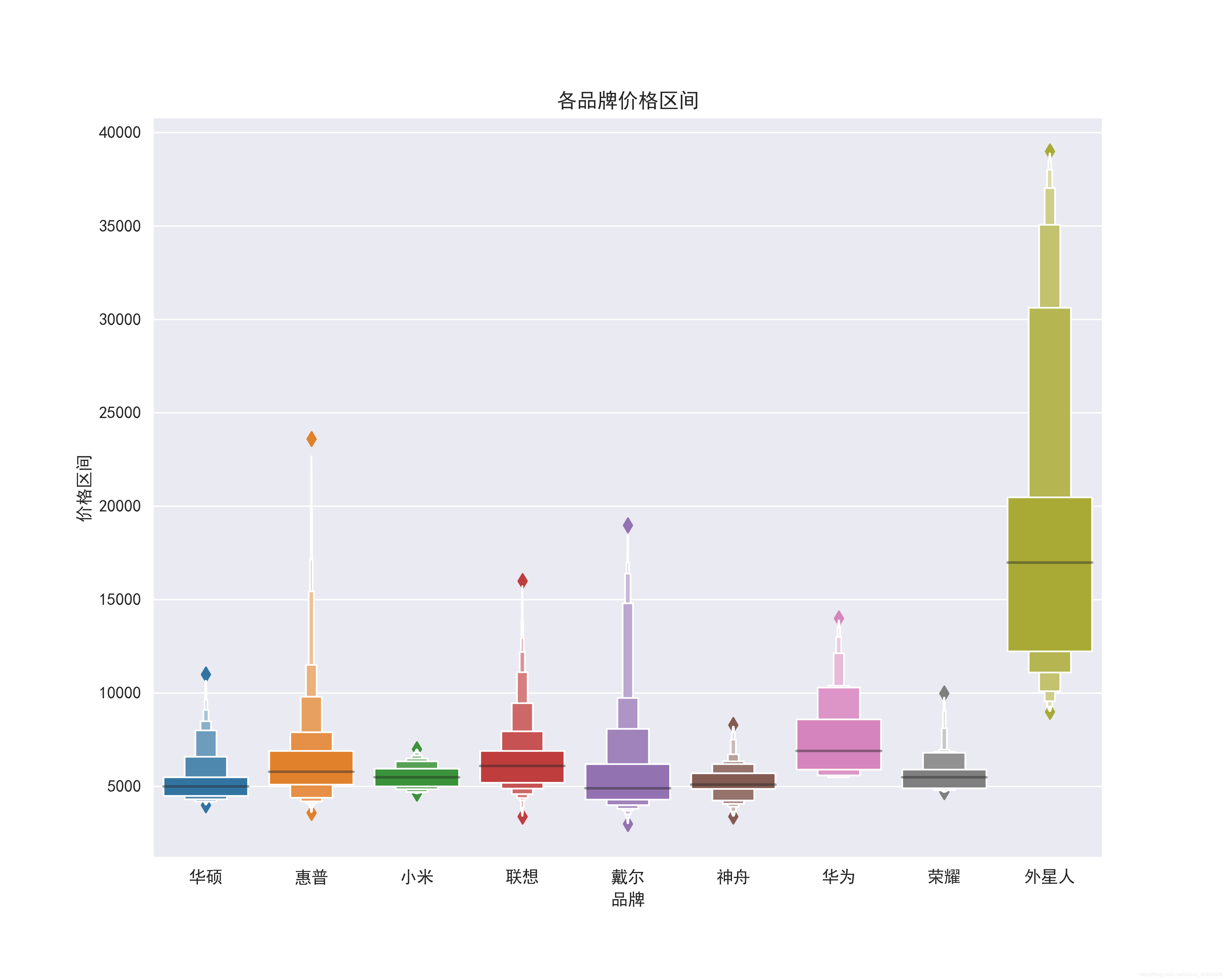
8.11 查看價格與評論數(shù)的關(guān)系
df_jp['computer_commit'] = df_jp['computer_commit'].astype('int64')
ax = sns.jointplot(x="computer_commit", y="computer_price", data=df_jp, kind="reg", truncate=False,color="m", height=10)
ax.fig.savefig('評論數(shù)與價格的關(guān)系.png')
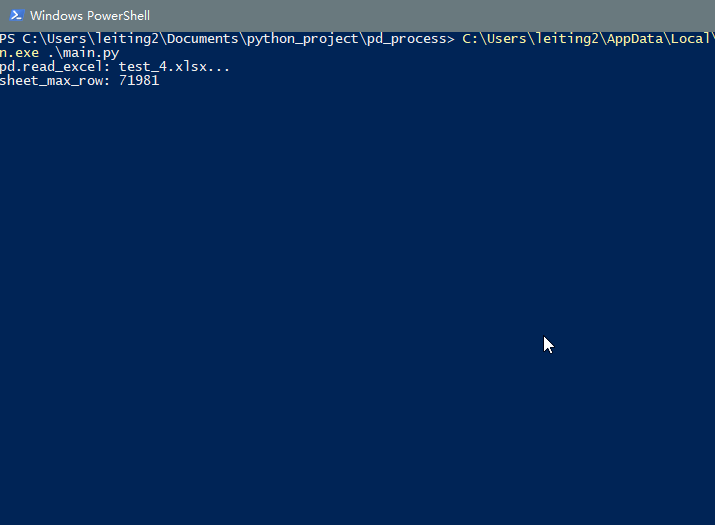
8.12 查看商品標題里出現(xiàn)的關(guān)鍵詞
import imageio
# 將特征轉(zhuǎn)換為列表
ls = df_jp['computer_title'].to_list()
# 替換非中英文的字符
feature_points = [re.sub(r'[^a-zA-Z\u4E00-\u9FA5]+',' ',str(feature)) for feature in ls]
# 讀取停用詞
stop_world = list(pd.read_csv('./百度停用詞表.txt', engine='python', encoding='utf-8', names=['stopwords'])['stopwords'])
feature_points2 = []
for feature in feature_points: # 遍歷每一條評論
words = jieba.lcut(feature) # 精確模式,沒有冗余.對每一條評論進行jieba分詞
ind1 = np.array([len(word) > 1 for word in words]) # 判斷每個分詞的長度是否大于1
ser1 = pd.Series(words)
ser2 = ser1[ind1] # 篩選分詞長度大于1的分詞留下
ind2 = ~ser2.isin(stop_world) # 注意取反負號
ser3 = ser2[ind2].unique() # 篩選出不在停用詞表的分詞留下,并去重
if len(ser3) > 0:
feature_points2.append(list(ser3))
# 將所有分詞存儲到一個列表中
wordlist = [word for feature in feature_points2 for word in feature]
# 將列表中所有的分詞拼接成一個字符串
feature_str = ' '.join(wordlist)
# 標題分析
font_path = r'./simhei.ttf'
shoes_box_jpg = imageio.imread('./home.jpg')
wc=wordcloud.WordCloud(
background_color='black',
mask=shoes_box_jpg,
font_path = font_path,
min_font_size=5,
max_font_size=50,
width=260,
height=260,
)
wc.generate(feature_str)
plt.figure(figsize=(10, 8), dpi=100)
plt.imshow(wc)
plt.axis('off')
plt.savefig('標題提取關(guān)鍵詞')
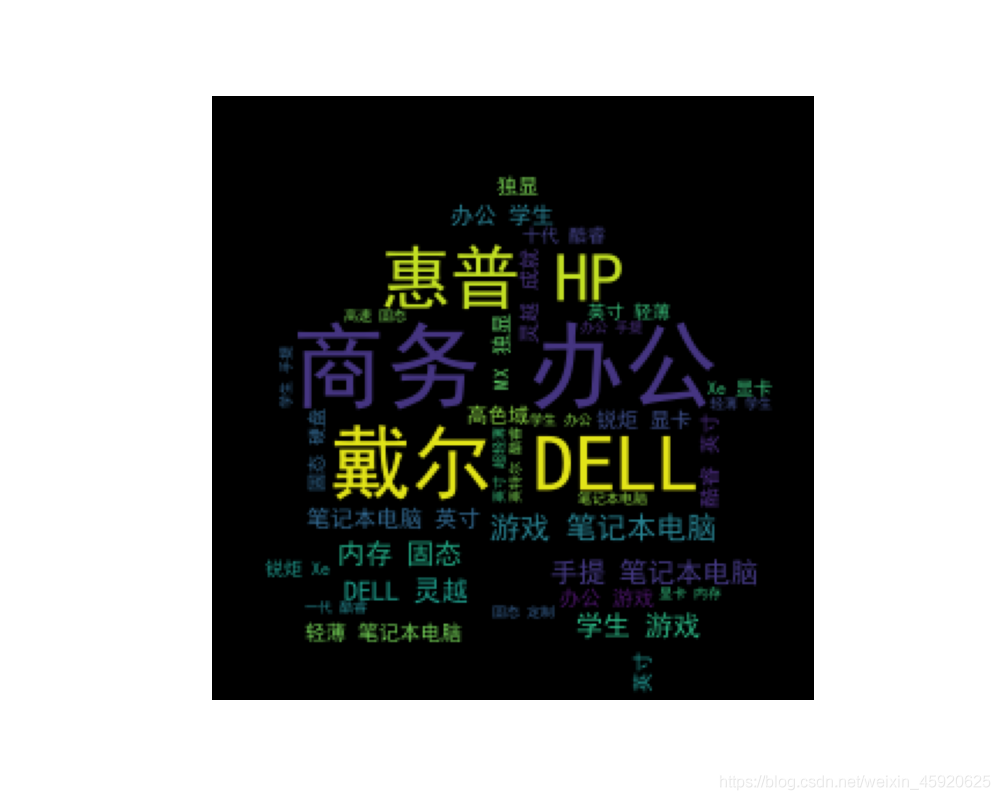
8.13 篩選價格在4000到5000,聯(lián)想品牌、處理器是i5、屏幕大小在15寸以上的數(shù)據(jù)并查看價格
df_jd_query = df_jp.loc[(df_jp['computer_price'] =5000) (df_jp['computer_price']>=4000) (df_jp['computer_p_shop']=="聯(lián)想") (df_jp['computer_intel']=="i5") (df_jp['computer_in']=="15.0英寸-15.9英寸"), :].copy()
plt.figure(figsize=(20, 10), dpi=100)
ax = sns.barplot(x='computer_sku', y='computer_price', data=df_jd_query)
ax.set_xlabel('聯(lián)想品牌SKU')
ax.set_ylabel('價格')
ax.set_title('酷睿i5處理器屏幕15寸以上各SKU的價格')
boxplot_fig = ax.get_figure()
boxplot_fig.savefig('酷睿i5處理器屏幕15寸以上各SKU的價格.png', dpi=400)
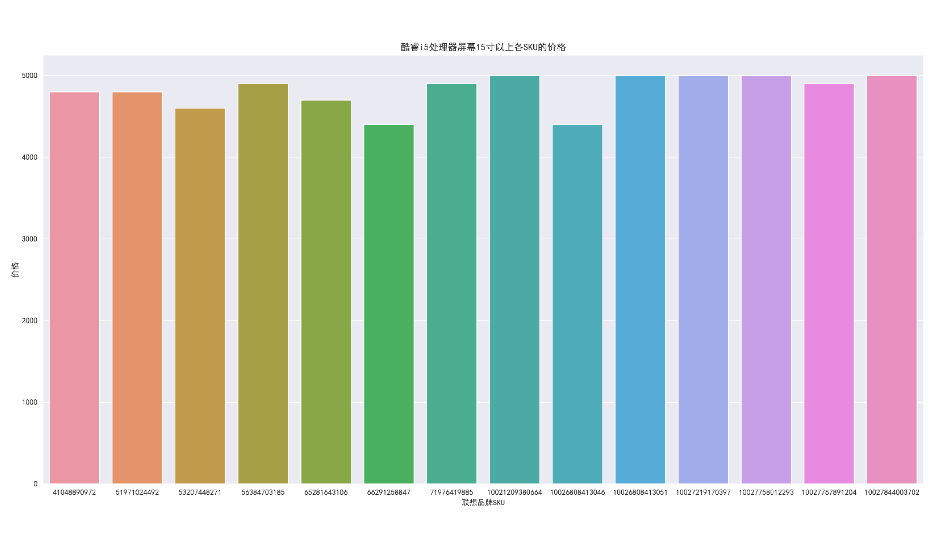
8.14 篩選價格在4000到5000,戴爾品牌、處理器是i7、屏幕大小在15寸以上的數(shù)據(jù)并查看價格
df_jp_daier = df_jp.loc[(df_jp['computer_price'] =5000) (df_jp['computer_price']>=4000) (df_jp['computer_p_shop']=="戴爾") (df_jp['computer_intel']=="i7") (df_jp['computer_in']=="15.0英寸-15.9英寸"), :].copy()
plt.figure(figsize=(10, 8), dpi=100)
ax = sns.barplot(x='computer_sku', y='computer_price', data=df_jp_daier)
ax.set_xlabel('戴爾品牌SKU')
ax.set_ylabel('價格')
ax.set_title('酷睿i7處理器屏幕15寸以上各SKU的價格')
boxplot_fig = ax.get_figure()
boxplot_fig.savefig('酷睿i7處理器屏幕15寸以上各SKU的價格.png', dpi=400)
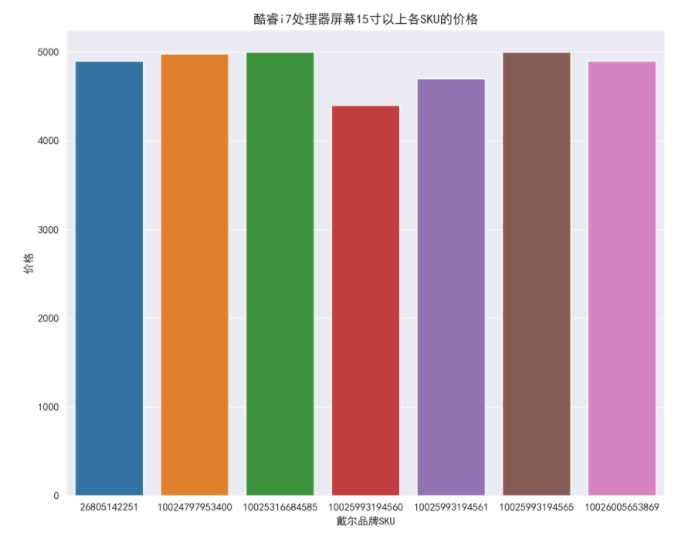
8.15 不同Intel處理器品牌的價格
plt.figure(figsize=(10, 8), dpi=100)
ax = sns.barplot(x='computer_p_shop', y='computer_price', data=df_jp, hue='computer_intel')
ax.set_xlabel('品牌')
ax.set_ylabel('價格')
ax.set_title('不同酷睿處理器品牌的價格')
boxplot_fig = ax.get_figure()
boxplot_fig.savefig('不同酷睿處理器品牌的價格.png', dpi=400)
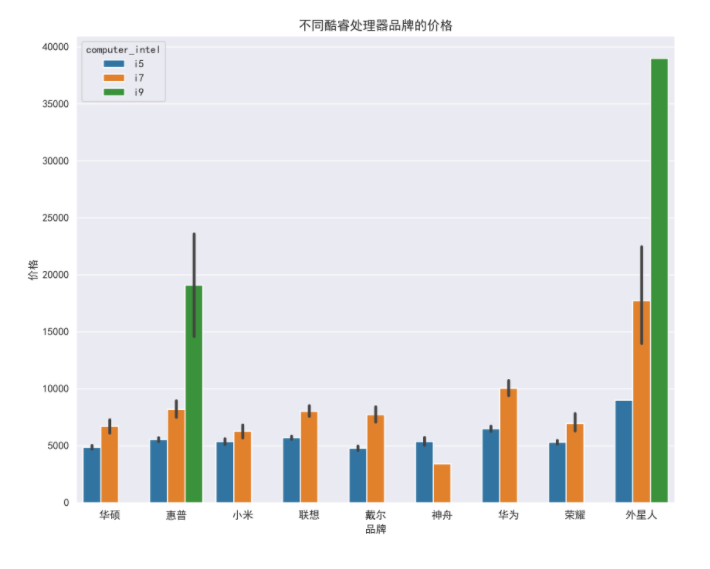
8.16 不同尺寸品牌的價格
plt.figure(figsize=(10, 8), dpi=100)
ax = sns.barplot(x='computer_p_shop', y='computer_price', data=df_jp, hue='computer_in')
ax.set_xlabel('品牌')
ax.set_ylabel('價格')
ax.set_title('不同尺寸品牌的價格')
boxplot_fig = ax.get_figure()
boxplot_fig.savefig('不同尺寸品牌的價格.png', dpi=400)
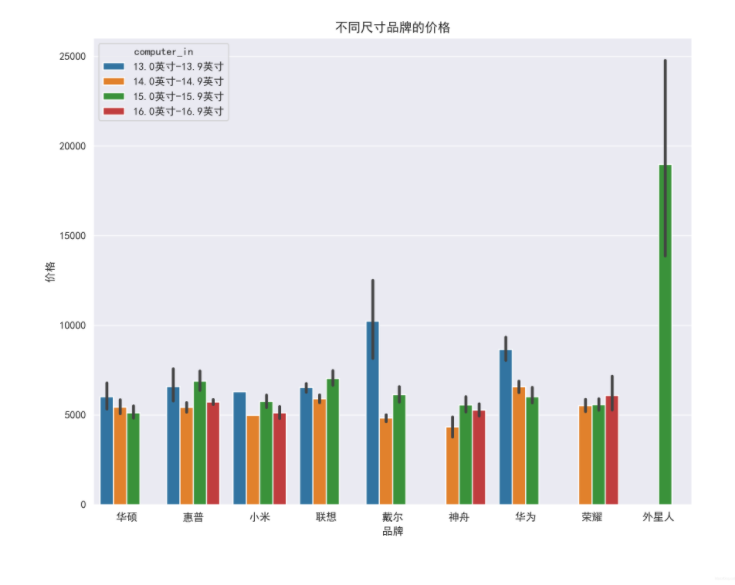
以上就是python基于scrapy爬取京東筆記本電腦數(shù)據(jù)并進行簡單處理和分析的詳細內(nèi)容,更多關(guān)于python 爬取京東數(shù)據(jù)的資料請關(guān)注腳本之家其它相關(guān)文章!
您可能感興趣的文章:- Android實現(xiàn)京東首頁效果
- JS實現(xiàn)京東商品分類側(cè)邊欄
- 利用JavaScript模擬京東按鍵輸入功能
- 仿京東平臺框架開發(fā)開放平臺(包含需求,服務(wù)端代碼,SDK代碼)