目錄
- 一、技術背景
- 二、MindSpore內置的損失函數
- 三、自定義損失函數
- 四、自定義其他算子
- 五、多層算子的應用
- 六、重定義reduction
一、技術背景
損失函數是機器學習中直接決定訓練結果好壞的一個模塊,該函數用于定義計算出來的結果或者是神經網絡給出的推測結論與正確結果的偏差程度,偏差的越多,就表明對應的參數越差。而損失函數的另一個重要性在于會影響到優化函數的收斂性,如果損失函數的指數定義的太高,稍有參數波動就導致結果的巨大波動的話,那么訓練和優化就很難收斂。一般我們常用的損失函數是MSE(均方誤差)和MAE(平均標準差)等。那么這里我們嘗試在MindSpore中去自定義一些損失函數,可用于適應自己的特殊場景。
二、MindSpore內置的損失函數
剛才提到的MSE和MAE等常見損失函數,MindSpore中是有內置的,通過net_loss = nn.loss.MSELoss()
即可調用,再傳入Model
中進行訓練,具體使用方法可以參考如下擬合一個非線性函數的案例:
# test_nonlinear.py
from mindspore import context
import numpy as np
from mindspore import dataset as ds
from mindspore import nn, Tensor, Model
import time
from mindspore.train.callback import Callback, LossMonitor
import mindspore as ms
ms.common.set_seed(0)
def get_data(num, a=2.0, b=3.0, c=5.0):
for _ in range(num):
x = np.random.uniform(-1.0, 1.0)
y = np.random.uniform(-1.0, 1.0)
noise = np.random.normal(0, 0.03)
z = a * x ** 2 + b * y ** 3 + c + noise
yield np.array([[x**2], [y**3]],dtype=np.float32).reshape(1,2), np.array([z]).astype(np.float32)
def create_dataset(num_data, batch_size=16, repeat_size=1):
input_data = ds.GeneratorDataset(list(get_data(num_data)), column_names=['xy','z'])
input_data = input_data.batch(batch_size)
input_data = input_data.repeat(repeat_size)
return input_data
data_number = 160
batch_number = 10
repeat_number = 10
ds_train = create_dataset(data_number, batch_size=batch_number, repeat_size=repeat_number)
class LinearNet(nn.Cell):
def __init__(self):
super(LinearNet, self).__init__()
self.fc = nn.Dense(2, 1, 0.02, 0.02)
def construct(self, x):
x = self.fc(x)
return x
start_time = time.time()
net = LinearNet()
model_params = net.trainable_params()
print ('Param Shape is: {}'.format(len(model_params)))
for net_param in net.trainable_params():
print(net_param, net_param.asnumpy())
net_loss = nn.loss.MSELoss()
optim = nn.Momentum(net.trainable_params(), learning_rate=0.01, momentum=0.6)
model = Model(net, net_loss, optim)
epoch = 1
model.train(epoch, ds_train, callbacks=[LossMonitor(10)], dataset_sink_mode=True)
for net_param in net.trainable_params():
print(net_param, net_param.asnumpy())
print ('The total time cost is: {}s'.format(time.time() - start_time))
訓練的結果如下:
epoch: 1 step: 160, loss is 2.5267093
Parameter (name=fc.weight, shape=(1, 2), dtype=Float32, requires_grad=True) [[1.0694231 0.12706374]]
Parameter (name=fc.bias, shape=(1,), dtype=Float32, requires_grad=True) [5.186701]
The total time cost is: 8.412306308746338s
最終優化出來的loss值是2.5,不過在損失函數定義不同的情況下,單純只看loss值是沒有意義的。所以通常是大家統一定一個測試的標準,比如大家都用MAE來衡量最終訓練出來的模型的好壞,但是中間訓練的過程不一定采用MAE來作為損失函數。
三、自定義損失函數
由于python語言的靈活性,使得我們可以繼承基本類和函數,只要使用mindspore允許范圍內的算子,就可以實現自定義的損失函數。我們先看一個簡單的案例,暫時將我們自定義的損失函數命名為L1Loss:
# test_nonlinear.py
from mindspore import context
import numpy as np
from mindspore import dataset as ds
from mindspore import nn, Tensor, Model
import time
from mindspore.train.callback import Callback, LossMonitor
import mindspore as ms
import mindspore.ops as ops
from mindspore.nn.loss.loss import Loss
ms.common.set_seed(0)
def get_data(num, a=2.0, b=3.0, c=5.0):
for _ in range(num):
x = np.random.uniform(-1.0, 1.0)
y = np.random.uniform(-1.0, 1.0)
noise = np.random.normal(0, 0.03)
z = a * x ** 2 + b * y ** 3 + c + noise
yield np.array([[x**2], [y**3]],dtype=np.float32).reshape(1,2), np.array([z]).astype(np.float32)
def create_dataset(num_data, batch_size=16, repeat_size=1):
input_data = ds.GeneratorDataset(list(get_data(num_data)), column_names=['xy','z'])
input_data = input_data.batch(batch_size)
input_data = input_data.repeat(repeat_size)
return input_data
data_number = 160
batch_number = 10
repeat_number = 10
ds_train = create_dataset(data_number, batch_size=batch_number, repeat_size=repeat_number)
class LinearNet(nn.Cell):
def __init__(self):
super(LinearNet, self).__init__()
self.fc = nn.Dense(2, 1, 0.02, 0.02)
def construct(self, x):
x = self.fc(x)
return x
start_time = time.time()
net = LinearNet()
model_params = net.trainable_params()
print ('Param Shape is: {}'.format(len(model_params)))
for net_param in net.trainable_params():
print(net_param, net_param.asnumpy())
class L1Loss(Loss):
def __init__(self, reduction="mean"):
super(L1Loss, self).__init__(reduction)
self.abs = ops.Abs()
def construct(self, base, target):
x = self.abs(base - target)
return self.get_loss(x)
user_loss = L1Loss()
optim = nn.Momentum(net.trainable_params(), learning_rate=0.01, momentum=0.6)
model = Model(net, user_loss, optim)
epoch = 1
model.train(epoch, ds_train, callbacks=[LossMonitor(10)], dataset_sink_mode=True)
for net_param in net.trainable_params():
print(net_param, net_param.asnumpy())
print ('The total time cost is: {}s'.format(time.time() - start_time))
這里自己定義的內容實際上有兩個部分,一個是construct函數中的計算結果的函數,比如這里使用的是求絕對值。另外一個定義的部分是reduction參數,我們從mindspore的源碼中可以看到,這個reduction函數可以決定調用哪一種計算方法,定義好的有平均值、求和、保持不變三種策略。
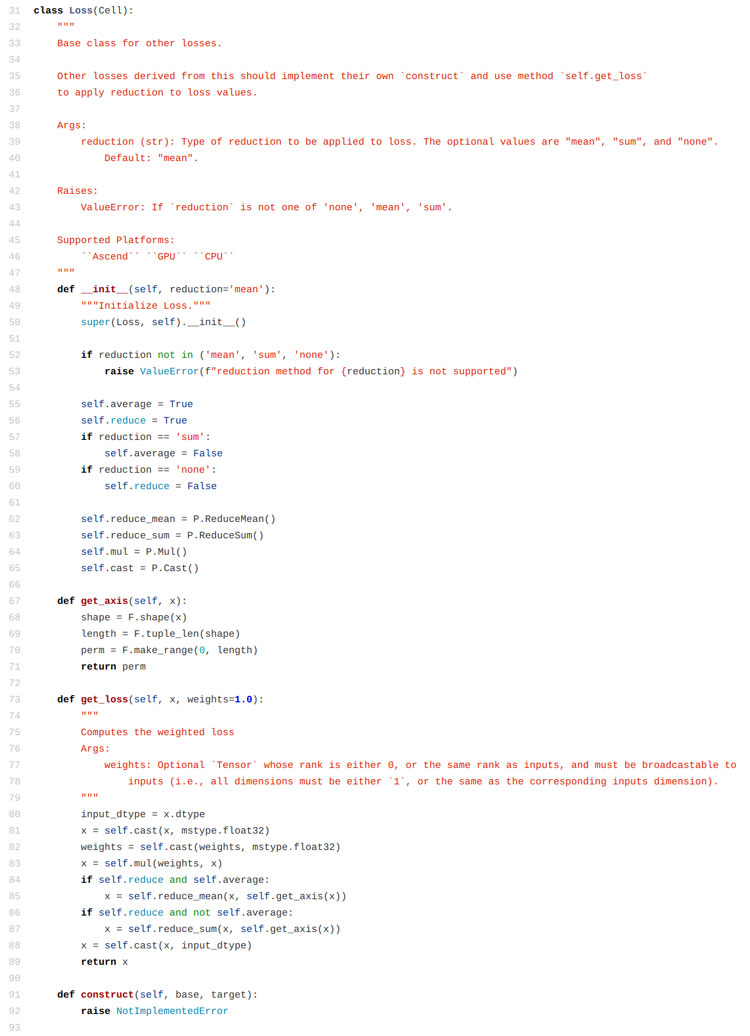
那么最后看下自定義的這個損失函數的運行結果:
epoch: 1 step: 160, loss is 1.8300734
Parameter (name=fc.weight, shape=(1, 2), dtype=Float32, requires_grad=True) [[ 1.2687287 -0.09565887]]
Parameter (name=fc.bias, shape=(1,), dtype=Float32, requires_grad=True) [3.7297544]
The total time cost is: 7.0749146938323975s
這里不必太在乎loss的值,因為前面也提到了,不同的損失函數框架下,計算出來的值就是不一樣的,小一點大一點并沒有太大意義,最終還是需要大家統一一個標準才能夠進行很好的衡量和對比。
四、自定義其他算子
這里我們僅僅是替換了一個abs的算子為square的算子,從求絕對值變化到求均方誤差,這里只是修改了一個算子,內容較為簡單:
# test_nonlinear.py
from mindspore import context
import numpy as np
from mindspore import dataset as ds
from mindspore import nn, Tensor, Model
import time
from mindspore.train.callback import Callback, LossMonitor
import mindspore as ms
import mindspore.ops as ops
from mindspore.nn.loss.loss import Loss
ms.common.set_seed(0)
def get_data(num, a=2.0, b=3.0, c=5.0):
for _ in range(num):
x = np.random.uniform(-1.0, 1.0)
y = np.random.uniform(-1.0, 1.0)
noise = np.random.normal(0, 0.03)
z = a * x ** 2 + b * y ** 3 + c + noise
yield np.array([[x**2], [y**3]],dtype=np.float32).reshape(1,2), np.array([z]).astype(np.float32)
def create_dataset(num_data, batch_size=16, repeat_size=1):
input_data = ds.GeneratorDataset(list(get_data(num_data)), column_names=['xy','z'])
input_data = input_data.batch(batch_size)
input_data = input_data.repeat(repeat_size)
return input_data
data_number = 160
batch_number = 10
repeat_number = 10
ds_train = create_dataset(data_number, batch_size=batch_number, repeat_size=repeat_number)
class LinearNet(nn.Cell):
def __init__(self):
super(LinearNet, self).__init__()
self.fc = nn.Dense(2, 1, 0.02, 0.02)
def construct(self, x):
x = self.fc(x)
return x
start_time = time.time()
net = LinearNet()
model_params = net.trainable_params()
print ('Param Shape is: {}'.format(len(model_params)))
for net_param in net.trainable_params():
print(net_param, net_param.asnumpy())
class L1Loss(Loss):
def __init__(self, reduction="mean"):
super(L1Loss, self).__init__(reduction)
self.square = ops.Square()
def construct(self, base, target):
x = self.square(base - target)
return self.get_loss(x)
user_loss = L1Loss()
optim = nn.Momentum(net.trainable_params(), learning_rate=0.01, momentum=0.6)
model = Model(net, user_loss, optim)
epoch = 1
model.train(epoch, ds_train, callbacks=[LossMonitor(10)], dataset_sink_mode=True)
for net_param in net.trainable_params():
print(net_param, net_param.asnumpy())
print ('The total time cost is: {}s'.format(time.time() - start_time))
關于更多的算子內容,可以參考下這個鏈接
(https://www.mindspore.cn/doc/api_python/zh-CN/r1.2/mindspore/mindspore.ops.html)中的內容,
上述代碼的運行結果如下:
epoch: 1 step: 160, loss is 2.5267093
Parameter (name=fc.weight, shape=(1, 2), dtype=Float32, requires_grad=True) [[1.0694231 0.12706374]]
Parameter (name=fc.bias, shape=(1,), dtype=Float32, requires_grad=True) [5.186701]
The total time cost is: 6.87545919418335s
可以從這個結果中發現的是,計算出來的結果跟最開始使用的內置的MSELoss結果是一樣的,這是因為我們自定義的這個求損失函數的形式與內置的MSE是吻合的。
五、多層算子的應用
上面的兩個例子都是簡單的說明了一下通過單個算子構造的損失函數,其實如果是一個復雜的損失函數,也可以通過多個算子的組合操作來進行實現:
# test_nonlinear.py
from mindspore import context
import numpy as np
from mindspore import dataset as ds
from mindspore import nn, Tensor, Model
import time
from mindspore.train.callback import Callback, LossMonitor
import mindspore as ms
import mindspore.ops as ops
from mindspore.nn.loss.loss import Loss
ms.common.set_seed(0)
def get_data(num, a=2.0, b=3.0, c=5.0):
for _ in range(num):
x = np.random.uniform(-1.0, 1.0)
y = np.random.uniform(-1.0, 1.0)
noise = np.random.normal(0, 0.03)
z = a * x ** 2 + b * y ** 3 + c + noise
yield np.array([[x**2], [y**3]],dtype=np.float32).reshape(1,2), np.array([z]).astype(np.float32)
def create_dataset(num_data, batch_size=16, repeat_size=1):
input_data = ds.GeneratorDataset(list(get_data(num_data)), column_names=['xy','z'])
input_data = input_data.batch(batch_size)
input_data = input_data.repeat(repeat_size)
return input_data
data_number = 160
batch_number = 10
repeat_number = 10
ds_train = create_dataset(data_number, batch_size=batch_number, repeat_size=repeat_number)
class LinearNet(nn.Cell):
def __init__(self):
super(LinearNet, self).__init__()
self.fc = nn.Dense(2, 1, 0.02, 0.02)
def construct(self, x):
x = self.fc(x)
return x
start_time = time.time()
net = LinearNet()
model_params = net.trainable_params()
print ('Param Shape is: {}'.format(len(model_params)))
for net_param in net.trainable_params():
print(net_param, net_param.asnumpy())
class L1Loss(Loss):
def __init__(self, reduction="mean"):
super(L1Loss, self).__init__(reduction)
self.square = ops.Square()
def construct(self, base, target):
x = self.square(self.square(base - target))
return self.get_loss(x)
user_loss = L1Loss()
optim = nn.Momentum(net.trainable_params(), learning_rate=0.01, momentum=0.6)
model = Model(net, user_loss, optim)
epoch = 1
model.train(epoch, ds_train, callbacks=[LossMonitor(10)], dataset_sink_mode=True)
for net_param in net.trainable_params():
print(net_param, net_param.asnumpy())
print ('The total time cost is: {}s'.format(time.time() - start_time))
這里使用的函數是兩個平方算子,也就是四次方的均方誤差,運行結果如下:
epoch: 1 step: 160, loss is 16.992222
Parameter (name=fc.weight, shape=(1, 2), dtype=Float32, requires_grad=True) [[0.14460069 0.32045612]]
Parameter (name=fc.bias, shape=(1,), dtype=Float32, requires_grad=True) [5.6676607]
The total time cost is: 7.253541946411133s
在實際的運算過程中,我們肯定不能夠說提升損失函數的冪次就一定能夠提升結果的優劣,但是通過多種基礎算子的組合,理論上說我們在一定的誤差允許范圍內,是可以實現任意的一個損失函數(通過泰勒展開取截斷項)的。
六、重定義reduction
方才提到這里面自定義損失函數的兩個重點,一個是上面三個章節中所演示的construct
函數的重寫,這部分實際上是重新設計損失函數的函數表達式。另一個是reduction
的自定義,這部分關系到不同的單點損失函數值之間的關系。舉個例子來說,如果我們將reduction
設置為求和,那么get_loss()
這部分的函數內容就是把所有的單點函數值加起來返回一個最終的值,求平均值也是類似的。那么通過自定義一個新的get_loss()
函數,我們就可以實現更加靈活的一些操作,比如我們可以選擇將所有的結果乘起來求積而不是求和(只是舉個例子,大部分情況下不會這么操作)。在python中要重寫這個函數也容易,就是在繼承父類的自定義類中定義一個同名函數即可,但是注意我們最好是保留原函數中的一些內容,在原內容的基礎上加一些東西,冒然改模塊有可能導致不好定位的運行報錯。
# test_nonlinear.py
from mindspore import context
import numpy as np
from mindspore import dataset as ds
from mindspore import nn, Tensor, Model
import time
from mindspore.train.callback import Callback, LossMonitor
import mindspore as ms
import mindspore.ops as ops
from mindspore.nn.loss.loss import Loss
ms.common.set_seed(0)
def get_data(num, a=2.0, b=3.0, c=5.0):
for _ in range(num):
x = np.random.uniform(-1.0, 1.0)
y = np.random.uniform(-1.0, 1.0)
noise = np.random.normal(0, 0.03)
z = a * x ** 2 + b * y ** 3 + c + noise
yield np.array([[x**2], [y**3]],dtype=np.float32).reshape(1,2), np.array([z]).astype(np.float32)
def create_dataset(num_data, batch_size=16, repeat_size=1):
input_data = ds.GeneratorDataset(list(get_data(num_data)), column_names=['xy','z'])
input_data = input_data.batch(batch_size)
input_data = input_data.repeat(repeat_size)
return input_data
data_number = 160
batch_number = 10
repeat_number = 10
ds_train = create_dataset(data_number, batch_size=batch_number, repeat_size=repeat_number)
class LinearNet(nn.Cell):
def __init__(self):
super(LinearNet, self).__init__()
self.fc = nn.Dense(2, 1, 0.02, 0.02)
def construct(self, x):
x = self.fc(x)
return x
start_time = time.time()
net = LinearNet()
model_params = net.trainable_params()
print ('Param Shape is: {}'.format(len(model_params)))
for net_param in net.trainable_params():
print(net_param, net_param.asnumpy())
class L1Loss(Loss):
def __init__(self, reduction="mean", config=True):
super(L1Loss, self).__init__(reduction)
self.square = ops.Square()
self.config = config
def construct(self, base, target):
x = self.square(base - target)
return self.get_loss(x)
def get_loss(self, x, weights=1.0):
print ('The data shape of x is: ', x.shape)
input_dtype = x.dtype
x = self.cast(x, ms.common.dtype.float32)
weights = self.cast(weights, ms.common.dtype.float32)
x = self.mul(weights, x)
if self.reduce and self.average:
x = self.reduce_mean(x, self.get_axis(x))
if self.reduce and not self.average:
x = self.reduce_sum(x, self.get_axis(x))
if self.config:
x = self.reduce_mean(x, self.get_axis(x))
weights = self.cast(-1.0, ms.common.dtype.float32)
x = self.mul(weights, x)
x = self.cast(x, input_dtype)
return x
user_loss = L1Loss()
optim = nn.Momentum(net.trainable_params(), learning_rate=0.01, momentum=0.6)
model = Model(net, user_loss, optim)
epoch = 1
model.train(epoch, ds_train, callbacks=[LossMonitor(10)], dataset_sink_mode=True)
for net_param in net.trainable_params():
print(net_param, net_param.asnumpy())
print ('The total time cost is: {}s'.format(time.time() - start_time))
上述代碼就是一個簡單的案例,這里我們所做的操作,僅僅是把之前均方誤差的求和改成了求和之后取負數。還是需要再強調一遍的是,雖然我們定義的函數是非常簡單的內容,但是借用這個方法,我們可以更加靈活的去按照自己的設計定義一些定制化的損失函數。上述代碼的執行結果如下:
The data shape of x is:
(10, 10, 1)
...
The data shape of x is:
(10, 10, 1)
epoch: 1 step: 160, loss is -310517200.0
Parameter (name=fc.weight, shape=(1, 2), dtype=Float32, requires_grad=True) [[-6154.176 667.4569]]
Parameter (name=fc.bias, shape=(1,), dtype=Float32, requires_grad=True) [-16418.32]
The total time cost is: 6.681089878082275s
一共打印了160個The data shape of x is...
,這是因為我們在劃分輸入的數據集的時候,選擇了將160個數據劃分為每個batch含10個元素的模塊,那么一共就有16個batch,又對這16個batch重復10次,那么就是一共有160個batch,計算損失函數時是以batch為單位的,但是如果只是計算求和或者求平均值的話,不管劃分多少個batch結果都是一致的。
以上就是詳解MindSpore自定義模型損失函數的詳細內容,更多關于MindSpore自定義模型損失函數的資料請關注腳本之家其它相關文章!
您可能感興趣的文章:- Python辦公自動化之教你用Python批量識別發票并錄入到Excel表格中
- Python基礎之注釋的用法
- 在Linux下使用命令行安裝Python